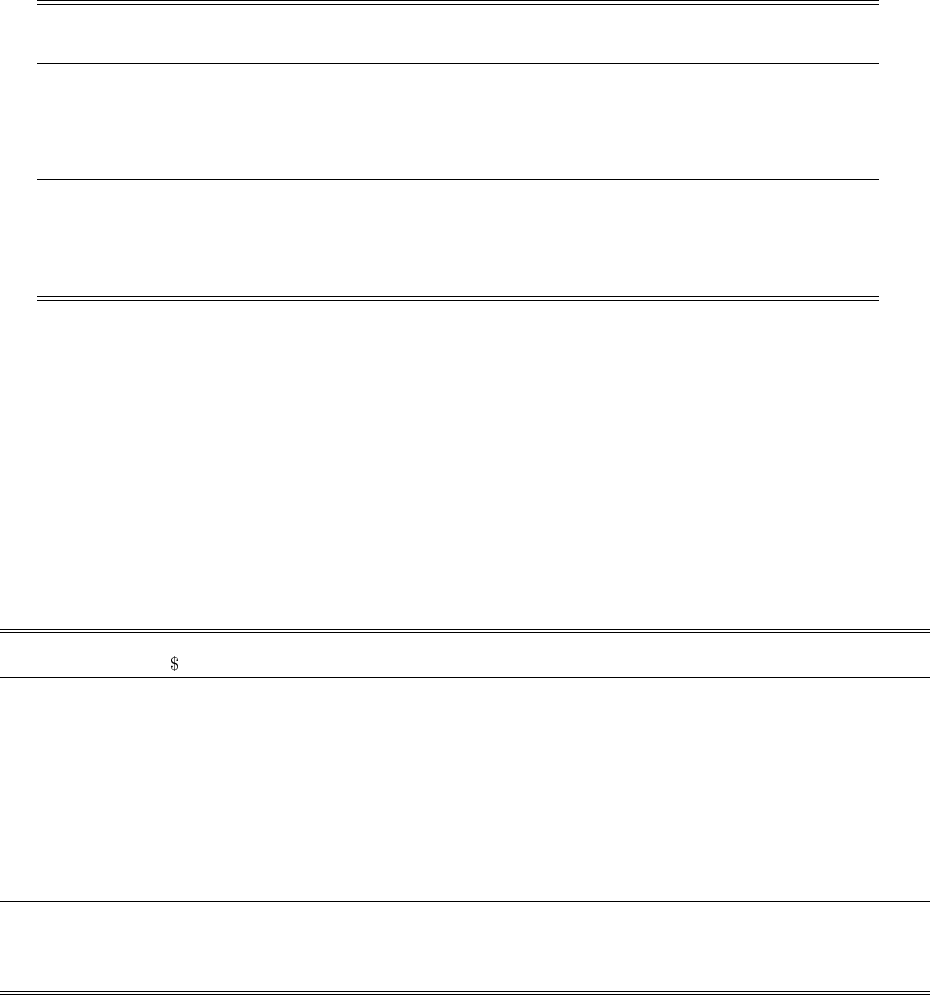
Table 12: Time to Close
Days 30+ Days 45+ Days 60+ Days
Mean 42.0 0.72 0.35 0.16
Treat*Post -2.0*** -0.034*** -0.032* -0.014
(0.54) (0.013) (0.019) (0.011)
Treat 1.6** 0.035** 0.025 -0.0012
(0.61) (0.014) (0.022) (0.013)
FE CBSA,Year CBSA,Year CBSA,Year CBSA,Year
Lender,Agency Lender,Agency Lender,Agency Lender,Agency
R-squared 0.29 0.22 0.22 0.20
N 1,132,834 1,132,834 1,132,834 1,132,834
* 0.10 ** 0.05 *** 0.01
Notes: Dependent variable is the number of days between a home under contract and the home closing
(column (1)), or indicator for if it took more than 45 days (column (2)), 60 days (column (3)), or 75 days
(column (4)) to close. Unit of observation is a loan matched to a home purchase. Treat*Post represents
the coefficient of interest and equals one if the lender and agency are a merged pair. Treat equals one
for any lender-agency pairs which ever merge. Standard errors are clustered at the agency level. ALl
columns include CBSA, Year, Lender, and Agency fixed effects.
Table 13: Borrower Characteristics
Amount LTV FICO 30 Days 60 Days 90 Days
Mean 254,484 87.00 736 14.2% 7.8% 5.8%
Treat*Post -8,213 -0.55 3.1* -0.0072 0.0051 0.0012
(5,923) (0.71) (1.8) (0.011) (0.0062) (0.0043)
Treat 2,692 0.28 -2.1 0.016 -0.0067 -0.0076
(6,431) (0.74) (2.1) (0.013) (0.0084) (0.0062)
FICO*LTV -0.000025*** -0.000022*** -0.000019***
(5.3e-07) (4.1e-07) (3.6e-07)
FICO Score 0.00053*** 0.00087*** 0.00083***
(0.000047) (0.000036) (0.000031)
LTV 0.020*** 0.017*** 0.015***
(0.00041) (0.00032) (0.00028)
FE CBSA,Year CBSA,Year CBSA,Year CBSA,Year CBSA,Year CBSA,Year
Lender,Agency Lender,Agency Lender,Agency Lender,Agency Lender,Agency Lender,Agency
R-squared 0.52 0.20 0.18 0.16 0.14 0.13
N 846,338 1,189,050 1,096,155 1,095,983 1,095,983 1,095,983
* 0.10 ** 0.05 *** 0.01
Notes: Dependent variable is loan amount (column (1)), loan-to-value ratio (column (2)), FICO (credit)
score at origination (column (3)), or indicator for if loan is ever 30 days delinquent (column (4)), 60 days
delinquent (column (5)), or 90 days delinquent (column (6)). Unit of observation is a loan matched to a
home purchase. Treat*Post represents the coefficient of interest and equals one if the lender and agency
are a merged pair. Treat equals one for any lender-agency pairs which ever merge. Standard errors are
clustered at the agency level.
55