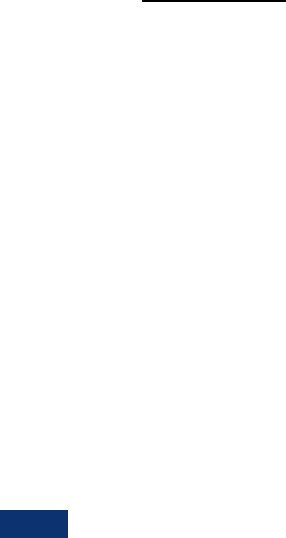
The Future of Computer Trading in Financial Markets
further below. Even if the effects were temporary and the original driving variables were to revert
to their long-term average values over time, some further irreversible events may have occurred,
such as nancial losses or even bankruptcies due to forced sales or to the triggering of penalty
clauses in contracts. At very high speeds, sensitivity may also be positively related to speed.
• Information: the existence of excessive nonlinear sensitivities can be due to informational issues: in
other words, who knows what and when. The information structure of a market has the potential
to increase or reduce market swings through a number of subtle, and sometimes contradictory,
effects. To illustrate this, academic studies have explored behaviour that arises in coordination games
with diffuse information
14
. In these game scenarios, agents coordinate to create a ‘bank-run’ on an
institution, a security or a currency if a given publicly observed signal is bad enough. Only very small
differences in the signal, say the number of write-offs of a bank, determine whether creditors run
or stay. With diffuse information, violent cascades of failures over an entire market system can be
triggered by such small events
15
.
• Endogenous risk: this term, commonplace in the nancial literature
16
, identies features wholly within
the nancial markets that lead in some situations to the sudden (due to the nonlinearities involved)
emergence of positive (i.e. mutually reinforcing) and pernicious feedback loops, whether market
participants are fully rational or otherwise
17
.
In the section that follows, a number of feedback loops that can contribute to endogenous risk are
explored. One of the reasons the sensitivities and feedback loops at very high speeds may look
qualitatively different from those at lower speeds, even if accelerated, lies in the fact that beyond
the limits of human response times, any feedback loops must be generated solely by robots
18
. It is
plausible that the properties of securities return time series at different frequencies are very similar to
each other through scaling
19
, at least as long as the frequency is low enough so that micro structure
effects do not come into the picture. This similarity may no longer hold beyond a critical frequency
at which only machines can react to each other. One of the reasons may be the discontinuity in the
set of possible feedback loops as one goes to very small scales and excludes human interactions
20
.
While ‘mini-ash crashes’ occur regularly at very small scales, they are mostly self-healing in a matter
of milliseconds and aggregate to noise without attracting much attention
21
. This does not mean that a
mini-ash crash of the feedback loop variety (as opposed to an execution algorithm simply splitting a
large trade and continuously buying or selling) could not form a chain reaction, gather momentum and
be visible at human scales also, or indeed lead human traders to feed into the loop.
14 See, for example, Carlsson & Van Damme (1993) and Morris & Shin (2002).
15 Information, sensitivity and speed interact. For instance, with lower tick sizes the frequency of order book updating increases
dramatically. Given possible and realistic latencies for traders, there would be inevitable information loss: no trader would at
any moment in time be able to have an accurate idea of the current state of the order book, let alone an accurate depiction
of multiple order books across trading venues. Very fast markets make concurrent precise order book information virtually
impossible and lead to trading decisions taken under a veil of incomplete knowledge, not only about the future but also about
the present. Trading strategies need to second-guess the state, possibly leading to over-reactions (see Zigrand (2005), for
a formal model). In that sense, speed inuences both information and sensitivity according to a U shape: perhaps, as with a
bicycle, both too low a speed and too high a speed lead to inefciencies and instabilities.
16 See Danielsson & Shin (2003), Danielsson et al. (2011) and Shin (2010).
17 See DR2, DR6, DR7, and DR9 (Annex D refers).
18 See DR6, DR7 and DR27 (Annex D refers). DR27 suggests a transition may occur at around 1000 microseconds.
19 This is the so-called characteristic of self-similarity, roughly saying for instance that the distribution of hourly returns is very
similar to the distribution of minute by minute returns when minute by minute returns are multiplied by the appropriate
scaling factor of 60 to a power H. For example in the Gaussian case H=1/2. In that sense, self-similarity means that short-dated
returns really are just a sped-up version of lower frequency returns, with no qualitative break in their statistical behaviour.
20 At sub-human scales all other AT activities also operate without direct human judgement, so one execution algorithm can
lead to many price-adjustment ticks in the same direction even without any feedback loops, leading to mini-ash crashes if
the order book is not concurrently replenished. The vast majority of such events heal themselves through limit order book
replenishment, leaving the crashes invisible at lower frequencies. If, on top of an execution algorithm at work, feedback loops
form at sub-human scales, then the dynamics become nonlinear and the crash may well be visible at human scales, unless
preemptive circuit breakers successfully kicked in.
74
21 Also see DR29 (Annex D refers). Most of the evidence in that review is based on US data.