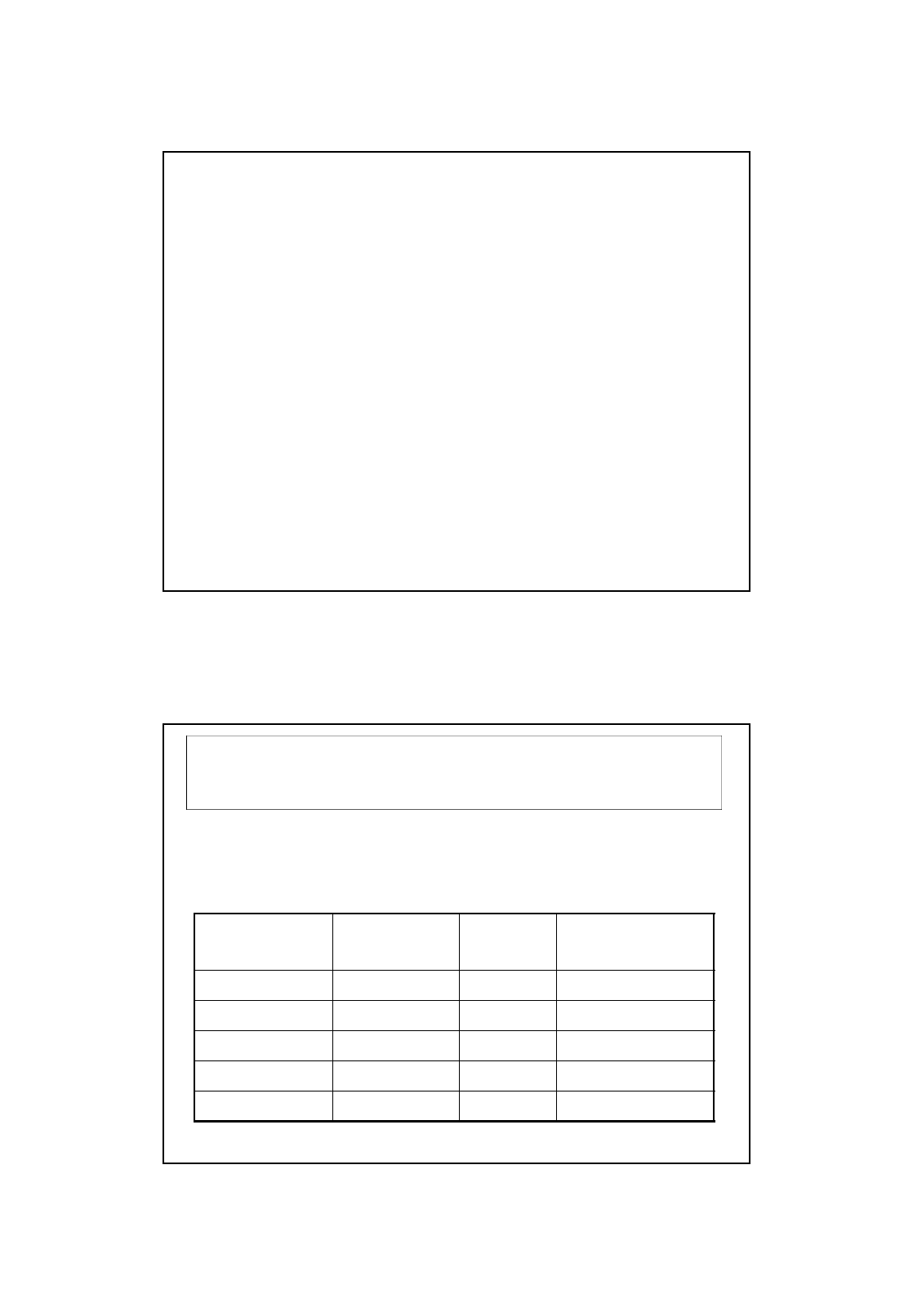
12
Two possible solutions:
1) Provide the unadjusted p-values.
Small ones (p<0.001) should be OK, since they should remain
even after allowing for several comparisons
2) Be cautious about 0.05<p<0.01
• Provide 97.5% confidence intervals for the effects sizes if there are,
say 2 or 3 endpoints, and 99% CIs for >3 endpoints
• These give a conservative range of true effect sizes.
• If a 99% CI still does not include the no effect value, then there is
likely to be a real effect
• If using 97.5% CI, then the p-value cut-off to determine statistical
significance should then be 0.025 (not 0.05 as is usual)
• If using 99% CI, then the p-value cut-off to determine statistical
significance should then be 0.01 (not 0.05 as is usual)
Endpoint
(all are measured
on a 0 to 100 scale)
Effect size (mean
difference), 99%
CI
Unadjusted
p-value
Possible conclusions
Global health status -0.6 (-4.0, +2.7) 0.62
Nausea & vomiting -1.9 (-4.4, +0.6) 0.048
Insomnia -10.0 (-14.5, -5.5) <0.0001
Constipation +10.6 (+6.2, +15.0) <0.0001
Financial difficulties +3.6 (-1.5, +8.8) 0.07
Note: For 99% CI, the p-value should be < 0.01 and not < 0.05
Example: quality of life measured in a trial comparing 2 treatments.
The survey has 25 items so each is an endpoint (5 are shown below), the survey is
administered several times per patient over time (i.e. repeated measures)
We could therefore have 25 separate (mixed modelling) results
Changing the confidence interval: an example